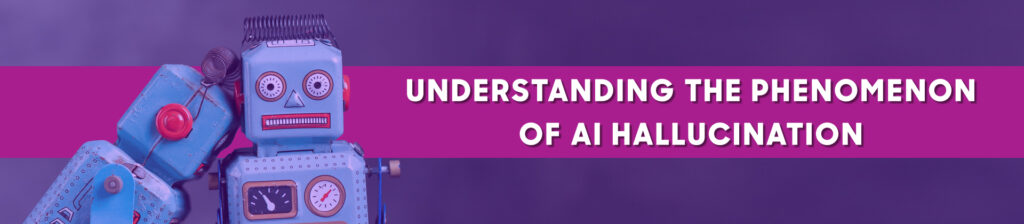
Exploring the Challenge of AI Hallucination
Artificial Intelligence (AI) has made significant strides in the past decade. It has enabled machines to learn and perform tasks that were once exclusively human, such as recognizing faces, translating languages, and driving cars. However, AI is not perfect, and one of the issues that has come to light is AI hallucination. AI hallucination is a phenomenon in which an AI model generates output that is not grounded in reality, but rather is a product of its internal biases or training data.
AI hallucination can take many forms, from generating images of nonexistent animals to misclassifying objects. In some cases, AI models can even generate text or speech that is completely fabricated. For example, in 2019, OpenAI released GPT-2, a language model that generated highly coherent and convincing text. However, it was also capable of generating fake news stories and misleading information.
AI hallucination is a result of the way that AI models are trained. Machine learning models learn from examples, and the quality of the output depends on the quality of the data used to train them. If the data contains biases or inaccuracies, the model will learn and replicate those biases. Furthermore, if the model is trained on a narrow set of data, it may not be able to generalize to new scenarios. This can lead to AI models generating hallucinations that are unrealistic or even harmful.
One example of AI hallucination is the case of Google’s DeepDream algorithm. DeepDream was originally developed as a tool for visualizing the features that an AI model is focusing on when analyzing an image. However, researchers soon discovered that they could use the algorithm to generate hallucinatory images by repeatedly feeding an image back into the model and amplifying certain features. The resulting images were surreal and otherworldly, but not grounded in reality.
Another example of AI hallucination is the case of image recognition algorithms misclassifying objects. In 2015, a group of researchers found that an AI model trained on a dataset of images that predominantly featured white people was more likely to misclassify images of people with darker skin tones. This is because the model had learned to associate certain features, such as facial hair or dark clothing, with the label “criminal.” As a result, it was more likely to misclassify images of people of color as criminals, even if they were not.
AI hallucination is not just a theoretical problem; it can have real-world consequences. For example, if an AI model misclassifies an object in a self-driving car, it could lead to an accident. Similarly, if an AI model generates fake news or propaganda, it could influence public opinion and even sway elections.
So, what can be done to address the problem of AI hallucination? One approach is to improve the quality of the data used to train AI models. This can be done by using more diverse datasets and by carefully selecting and curating the data. Another approach is to use adversarial training, in which a model is trained to recognize and reject hallucinations generated by other models. Additionally, researchers are exploring ways to incorporate human oversight and feedback into the training process, to ensure that the model is not generating output that is harmful or misleading.
In conclusion, AI hallucination is a significant problem within AI models. It is a result of the biases and limitations inherent in machine learning, and can have real-world consequences. Addressing this problem will require a multifaceted approach that involves improving the quality of data, using adversarial training, and incorporating human oversight into the training process. Ultimately, the goal is to create AI models that are not just accurate, but also grounded in reality and aligned with human values.
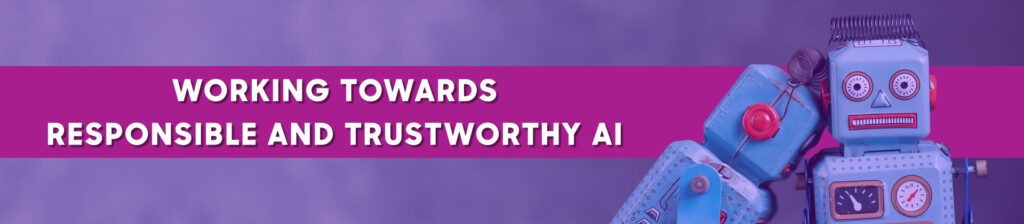
Addressing the Problem of AI Hallucination
To deal with the problem of AI hallucination, there are several actions we can take:
1. Improve the quality of data: As mentioned earlier, the quality of data used to train AI models has a significant impact on their performance. Therefore, it is essential to use diverse, high-quality datasets to train AI models that are less likely to generate hallucinations.
2. Incorporate human oversight: One way to reduce the risk of AI generating hallucinations is to incorporate human oversight into the training and deployment process. Humans can provide feedback and guidance to the AI model, identify errors, and correct them.
3. Use adversarial training: Adversarial training is a technique that involves training a model to recognize and reject hallucinations generated by other models. By doing this, AI models can learn to recognize and avoid generating hallucinations.
4. Promote transparency and accountability: It is essential to have transparency and accountability in the development and deployment of AI models. This includes transparency about the data used to train the models and the decision-making processes involved in their development. It also includes accountability for the performance of the models and their impact on society.
5. Educate the public: Educating the public about the potential risks and benefits of AI is essential to promoting responsible development and deployment of AI models. This includes educating individuals about the limitations of AI and the potential for AI to generate hallucinations.
Dealing with the problem of AI hallucination requires a concerted effort by both developers and users of AI models. By improving the quality of data, incorporating human oversight, using adversarial training, promoting transparency and accountability, and educating the public, we can work towards creating AI models that are reliable, trustworthy, and aligned with human values.